Exploring Stochastic Processes in Finance
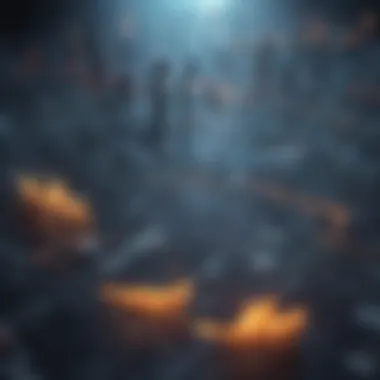
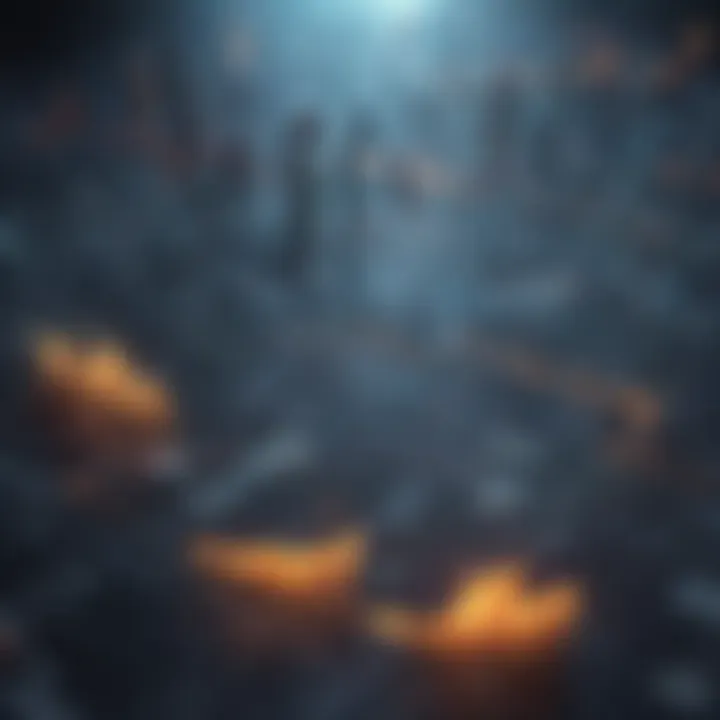
Intro
In the vast ocean of financial theory and practice, stochastic processes emerge as a crucial life raft, guiding investors and financial professionals through the unpredictable waves of market behavior. Essentially, stochastic processes allow us to model systems that evolve over time where uncertainty plays a significant role. From stock price movements to interest rate changes, understanding these processes can serve as a compass in navigating the myriad complexities faced by investors today.
In this article, we embark on a journey to dissect the essence of stochastic processes. We will delve into not only the foundational principles driving these models but also their applications across various domains, particularly in finance. The interplay of randomness and predictability yields insights that could transform one's investment strategy. Whether you are a seasoned investor, a finance student, or a professional advisor, the content of this piece aims to enrich your knowledge and arm you with the tools needed to grapple with the inherent uncertainties of the market.
By examining stochastic models and their implications, financial enthusiasts will develop a deeper appreciation for both the art and science behind making informed investment decisions. Are you ready to unravel the intricate tapestry of randomness in financial analysis? Let's dive in.
Preamble to Stochastic Processes
Stochastic processes stand as a cornerstone in modern finance, engineering, and numerous scientific disciplines. Understanding these processes is key for grasping how systems evolve in the presence of uncertainty. The beauty of stochastic processes lies in their ability to model randomness in a structured way, enabling predictions and analyses that would otherwise be unattainable. This introduction aims to highlight the importance of stochastic processes, their relevance, and the benefits they bring to various fields.
A stochastic process is, essentially, a collection of random variables. These variables could represent anything from stock prices fluctuating over time to the variation in temperatures across seasons. By studying these processes, one can gain insights into patterns of behavior and make informed decisions based on probabilities. For investors, this means understanding the market’s unpredictable nature, creating strategies that statistically mitigate risks and enhance returns.
Key Benefits of Understanding Stochastic Processes
- Risk Assessment: Investors can better evaluate the likelihood of adverse events affecting their portfolios.
- Prediction of Future Movements: By analyzing historical stochastic data, one can form expectations about future market behaviors.
- Strategic Planning: Stochastic models help in devising plans that incorporate variability, optimizing responses to uncertain conditions.
- Interdisciplinary Applications: The principles of stochastic processes are applicable across various domains, from biology to telecommunications, thus enhancing its importance.
Considerations About Stochastic Processes
It’s essential to recognize a few points regarding stochastic processes:
- Non-Deterministic: Unlike deterministic models, outcomes in stochastic processes are not fixed but random, making intuition alone an unreliable guide.
- Mathematical Complexity: Engaging with stochastic processes often requires a robust understanding of mathematical principles, particularly in probability theory.
- Dynamic Systems: These processes often involve continuous change, necessitating ongoing observations and adaptations in strategy.
As we delve deeper, we will clarify what stochastic processes mean, tracing their historical roots and how they've evolved into indispensable tools in many disciplines.
"Success in strategy comes from a blend of analytically understanding randomness and predictability."
In summary, the study of stochastic processes is not just an academic pursuit; it's a practical endeavor that enriches our understanding of systems plagued by uncertainty. As we advance through this piece, we will unpack the theoretical frameworks and practical applications that underscore their significance in today’s world.
Mathematical Foundations
Grasping the mathematical foundations of stochastic processes can feel like deciphering a coded message. It's essential for anyone venturing into this field, especially for those who aim to apply these concepts in finance and beyond. The importance of these foundations lies in how they serve as a building block for understanding the unpredictable nature of these processes. The underlying mathematics provides clarity and structure, allowing deeper insights into randomness, variability, and uncertainty in various applications.
To start off, an intuitive grasp of probability theory is imperative. Probability serves as the bedrock, equipping us with the tools to quantify uncertainty. By learning to navigate through the intricacies of probability distributions and random variables, individuals are better prepared to implement stochastic models effectively. What's more, familiarity with statistical concepts enhances analytical skills, essential for evaluating financial risks or potential returns. With a robust mathematical framework in place, individuals can draw meaningful conclusions from data, ultimately informing strategic decision-making.
Probability Theory Basics
Probability theory is not just about rolling dice or flipping coins. It extends far and wide, influencing various fields, including finance. In essence, it deals with the analysis of random phenomena. By defining events and probabilities, it lays the groundwork to comprehend more complex concepts later on.
Some key components include:
- Random Variables: These are numerical outcomes of random processes. They are divided into discrete and continuous types, each useful in different applications.
- Probability Distributions: Understanding how probabilities are assigned to different outcomes helps in modeling uncertain events. This can be crucial in anticipating financial market fluctuations.
- Expectation and Variance: The expected value offers a way to summarize a random variable, while variance measures the spread of the outcomes. This is particularly critical in risk assessment and management in financial contexts.
A good grasp of these components enables analysts to construct more complex models, illuminating the unpredictability found within financial markets. It also fosters a deeper understanding of various stochastic processes that emerge in real-life scenarios.
Key Definitions and Notations
When delving into the realm of stochastic processes, having clarity on definitions and notations is indispensable. It's akin to having a map before embarking on a journey. Here are some fundamental terms that every budding financial analyst should have at their fingertips:
- Stochastic Process: A collection of random variables representing the evolution of a system over time. They can reflect real-world phenomena such as stock prices or interest rates.
- State Space: This refers to the set of all possible states in which a process can exist. Formulating this accurately is crucial for modeling outcomes.
- Transition Probabilities: These are values indicating the likelihood of moving from one state to another in a Markov process.
- Martingales: A particular class of stochastic processes where the expected future value, given all past information, remains equal to the current value. These concepts are integral to the theory of financial modeling.
The way you define a process shapes your approach to understanding it. A sound grasp of the definitions can reveal the nuances that may otherwise go unnoticed.
Types of Stochastic Processes
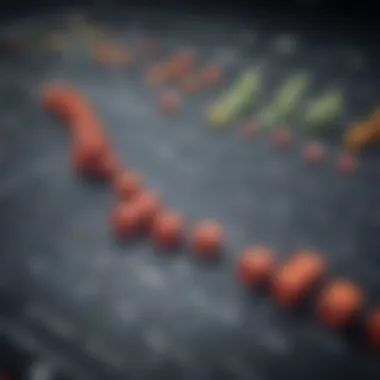
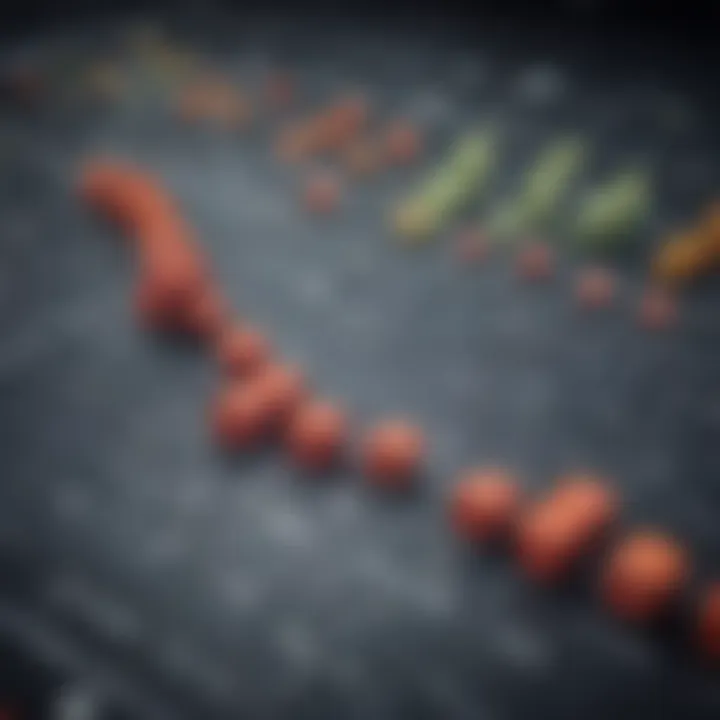
Understanding the types of stochastic processes is crucial for anyone looking to navigate the complex terrain of randomness and uncertainty inherent in various fields. Each type has its own unique characteristics and applications, making it essential for investors, finance professionals, and educators to grasp these distinctions. Here, we explore three key types of stochastic processes that are foundational to real-world applications: discrete versus continuous processes, Markov chains, and Brownian motion.
Discrete vs. Continuous Processes
In the realm of stochastic processes, one of the fundamental distinctions is between discrete and continuous processes. A discrete process, as the name suggests, consists of outcomes that occur at separate points in time and can typically be counted. Think of a traditional coin toss or the number of calls received at a call center during a specific hour; these examples fall into the discrete category. Each result is distinct and can be quantified easily, making the analysis straightforward.
On the other hand, continuous processes involve outcomes that can take on any value within a given range, often modeled over intervals of time. A prime example is the temperature readings throughout a day. Unlike the discrete framework, continuous processes provide a richer context for examining trends and variations that emerge over time. The choice between using discrete or continuous processes depends heavily on the nature of the data and the underlying questions being posed.
Key considerations in choosing between discrete and continuous processes include:
- Nature of the data: Is it countable or can it take on infinite values?
- Application context: How do the choices impact the models being created and analyzed?
- Complexity and computational feasibility: Continuous processes often require more sophisticated mathematical tools.
Markov Chains
Markov chains represent another critical aspect of stochastic processes. They embody the principle of memorylessness, meaning the future state of the process depends only on its current state, not on preceding states. To put it simply, it's like driving on a highway; your next move depends solely on the position you're at now, not on the journey that got you there.
Markov chains have found extensive applications in various fields such as finance, where they help in predicting stock movements. By creating a model based on current stock conditions, analysts can estimate possible future prices. The strength of Markov chains lies in their simplicity and foundational nature, making them a popular choice for modeling a variety of random processes.
Advantages of using Markov chains:
- Simplifies complex decision processes.
- Facilitates analysis through transition matrices, which provide clear pathways for movement between states.
- Applications range from finance, queuing theory, to genetics.
Brownian Motion
Brownian motion is often cited as a quintessential example of a continuous stochastic process. Named after the botanist Robert Brown, who first described the erratic movement of particles suspended in fluid, Brownian motion is crucial in fields like physics and finance. In finance, it serves as a model for the unpredictable nature of asset prices.
The characteristics of Brownian motion make it a valuable tool in quantitative finance. It captures the idea that asset prices move in a random walk pattern, incorporating both upward and downward trends with little predictability. Analysts utilize models like the Black-Scholes option pricing model, which is grounded in Brownian motion, to evaluate options and make investment decisions.
Notable features of Brownian motion include:
- Continuous paths that exhibit nowhere differentiable changes.
- The concept of a drift and volatility that can be modeled mathematically.
- Deep relevance in derivatives pricing and risk management strategies.
"Understanding the types of stochastic processes allows investors and practitioners to better navigate the unpredictabilities of the financial landscape and make informed decisions."
In summary, the types of stochastic processes form the backbone of understanding randomness across various domains. Whether one is tracking discrete outcomes or continuous changes, or leaning on structures like Markov chains and Brownian motion, knowledge of these processes equips individuals with the tools necessary to tackle uncertainty head-on.
Applications of Stochastic Processes
Stochastic processes find their footing in a variety of fields, reflecting their adaptability and significance. The idea of randomness isn’t just an abstract concept; it is woven into the very fabric of many real-world applications. Through stochastic modeling, professionals can gain insights into unpredictability while maintaining a semblance of control over their decisions. As we delve into specific domains, it's essential to appreciate how stochastic applications feed into broader economic and technological frameworks.
Finance and Investment
Finance and investment are perhaps the most prominent fields where the principles of stochastic processes are put to work. In this domain, professionals frequently encounter uncertainty, whether it originates from market fluctuations or investor behavior.
One key element is the Black-Scholes model, which applies stochastic differential equations to estimate the pricing of options. This model allows traders to gauge the expected volatility of stocks, giving them a clearer roadmap for making investment decisions. By employing such models, investors can better navigate the stormy seas of the financial market. The key is in understanding both the predictability and chaos that exist side by side.
Another important aspect is risk assessment. In finance, risk isn't merely about potential loss—it's about quantifying that risk in a sensible manner. Using stochastic processes, analysts can simulate various market scenarios, fleshing out potential outcomes based on historical data. The valuation of derivatives, portfolio management, and even capital allocation heavily rely on these stochastic insights.
Economics and Risk Management
Shifting gears to economics and risk management, stochastic processes serve as a vital backbone for policy formulation and economic modeling. In this context, decision-makers often find themselves grappling with uncertainty, be it in economic forecasting, assessing fiscal policies, or managing national budgets.
Models such as Markov chains help economists analyze various states of an economy over time, offering insights into possible future behaviors based on current conditions. For instance, understanding how consumer behavior changes in response to interest rates can be modeled with these chains, allowing policymakers to make data-driven decisions.
Additionally, risk management features prominently in insurance sectors where stochastic models predict claims and prime rates. When assessing risks, assessing randomness inherent in claim frequency and severity helps insurance firms to set more accurate premium rates, resulting in a more sustainable business model.
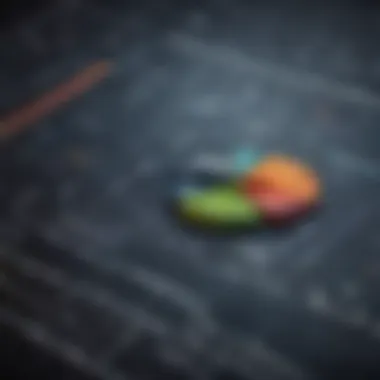
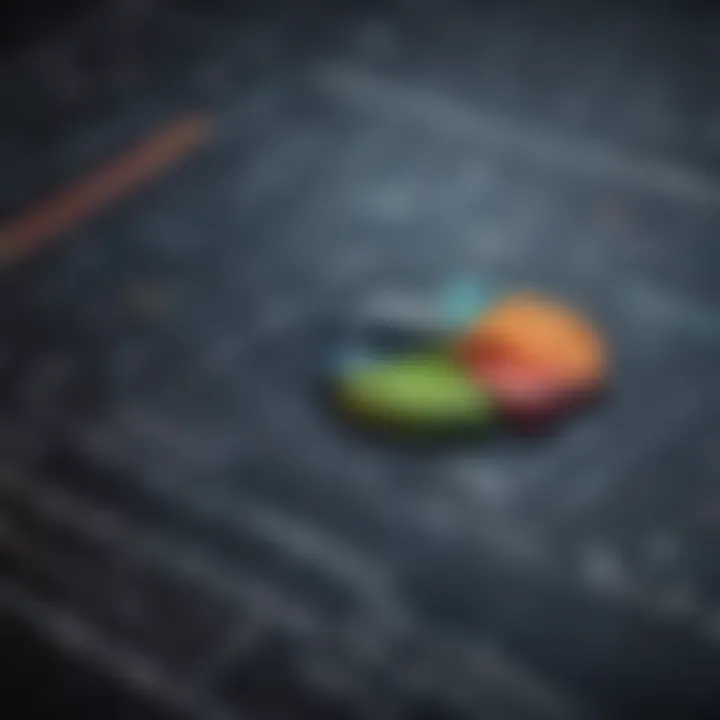
Engineering and Systems Reliability
In the realm of engineering and systems reliability, stochastic processes illuminate the pathways through which engineers can design resilient systems. Here, randomness may stem from material failures or unpredictable environmental events. Predictive maintenance and reliability engineering rely on stochastic models to forecast failures before they occur based on statistical data.
Through simulation techniques, engineers can analyze how systems respond under varying conditions. For instance, Monte Carlo simulations enable the assessment of risk in project management and systems reliability, giving engineers a clearer picture of potential pitfalls.
Moreover, reliability-based design optimization employs stochastic processes to ensure systems meet specific performance criteria over their life cycle. This methodological approach enhances both the durability and efficacy of engineering solutions.
In essence, the application of stochastic processes across industries is not merely about solving problems but understanding the randomness inherent in our world.
Beyond simply providing tools for analysis, stochastic models create a dialogue between data and decision-making, equipping professionals across different sectors with a robust framework for tackling uncertainty calmly and effectively.
Stochastic Models in Financial Analysis
In the realm of finance, stochastic models stand as pivotal tools for understanding market behavior and crafting investment strategies. These models, rooted in the principles of probability and randomness, enable analysts and investors to navigate the tumultuous waters of financial markets with a more nuanced perspective. By recognizing that prices and returns are often unpredictable, these models allow for a more sophisticated interpretation of data and trends.
Emphasizing the significance of stochastic models in financial analysis, it is crucial to recognize several specific elements and benefits:
- Risk Assessment: Stochastic models provide frameworks for quantifying uncertainty. This is especially vital for investors who wish to grasp the level of risk associated with different investments.
- Dynamic Pricing: Financial instruments such as options and futures rely heavily on stochastic processes for valuation. These models account for the inherent volatility of the market, ensuring more accurate pricing.
- Strategic Planning: Investors can use these models to simulate various scenarios, aiding in decision-making. By understanding potential outcomes based on different market conditions, they can devise informed strategies.
While the advantages of stochastic models are evident, they also come with considerations that practitioners should keep in mind. For example:
- Complexity: The mathematical underpinnings can be intricate, requiring a solid grounding in both finance and mathematics to apply effectively.
- Assumptions: Some models make assumptions that might not always hold in reality, which could lead to miscalculations. Analysts must be diligent in evaluating the assumptions of any stochastic model they employ.
"The world of finance is indeed a dance between randomness and predictability, with stochastic models revealing intricate patterns within the chaos."
An example of practical usage can be highlighted through the application of stochastic differential equations for pricing options, which is elaborated in the next section.
Pricing Options with Stochastic Differential Equations
When it comes to pricing options, stochastic differential equations (SDEs) play a critical role. The well-known Black-Scholes model, for instance, employs these equations to determine the value of options based on various factors such as the underlying asset price, time, and volatility. By introducing randomness into the pricing mechanism, SDEs allow for a more realistic reflection of market behavior, accounting for fluctuations that are characteristic of financial instruments.
The beauty of using SDEs lies in their ability to factor in volatility as a dynamic component, rather than static. Investors can thus derive options prices that more accurately represent the underlying risks and uncertainties inherent in the market.
Portfolio Management Strategies
In portfolio management, stochastic models serve as essential aids in creating robust investment strategies. By utilizing simulations such as Monte Carlo methods, investors can assess how different asset allocations might perform under varying market conditions. This enables them to understand not just potential returns, but also the risks associated with those returns.
Employing stochastic models allows portfolio managers to analyze:
- Diversification Benefits: By modeling various scenarios, managers can identify asset allocations that minimize risk while maximizing potential returns.
- Real-Time Adjustments: Stochastic models facilitate ongoing adjustments based on evolving market conditions, which is crucial in maintaining a competitive edge.
- Stress Testing: With the help of these models, portfolio managers can stress test their strategies under extreme market conditions to gauge their resilience.
The insightful application of stochastic models in financial analysis equips investors and professionals alike with a nuanced understanding of the unpredictability that pervades financial markets. It underscores a critical shift in perspective—from merely seeking predictability to embracing the uncertainty that characterizes financial returns. As we move further into an era defined by complex financial instruments and rapid market shifts, the importance of stochastic models cannot be overstated.
Challenges and Limitations of Stochastic Modeling
Stochastic modeling presents a maze of opportunities in understanding complex systems influenced by randomness. Even so, it’s not all sunshine and rainbows. This section digs deep into the challenges and limitations inherent in the world of stochastic processes, allowing investors and finance professionals to recognize the intricacies involved in predictive modeling.
Understanding Randomness
At the heart of stochastic processes lies randomness—an element that can feel like a double-edged sword. To make sense of a randomly fluctuating environment, investors often employ models that assume certain probabilities. But randomness isn't a mere backdrop; it can lead to erratic outcomes that even the best models struggle to accommodate. Here lies the first challenge: how does one accurately grasp randomness?
Consider stock prices which move seemingly without rhyme or reason. A strong earnings report may send shares skyrocketing one day, while negative headlines can tank them the next. The underlying unpredictability can baffle even seasoned analysts. Therefore, while stochastic models strive for precision in depicting randomness, they often fail to capture its wild spirit.
"The only thing predictable about randomness is that it's unpredictable."
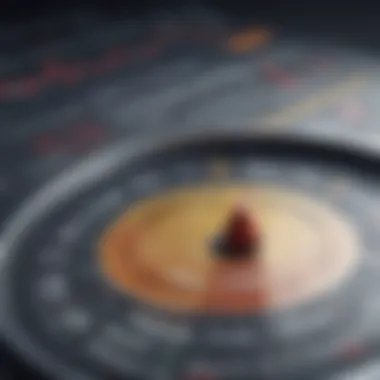
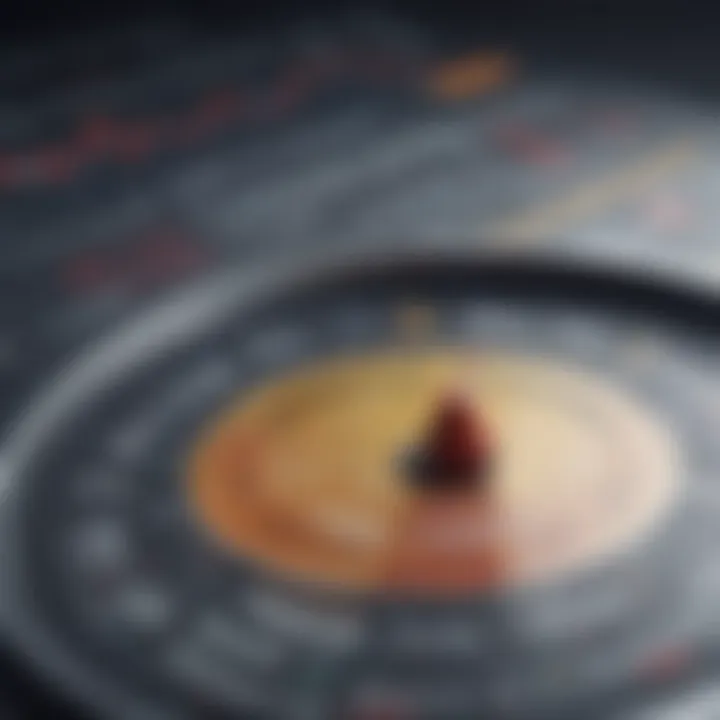
By delving deeper, we see randomness manifests in various forms—structural, parametric, or even measurement errors. Each has its own implications on forecasts and predictions. For example, structural randomness arises when the underlying relationships in data change unexpectedly, rendering previous models obsolete. Thus, while stochastic models can yield powerful insights, understanding the nuances of randomness is crucial to avoid pitfalls.
Model Assumptions and Their Impact
Another layer of complexity is added through model assumptions. Every stochastic model comes with its own set of assumptions that guide the analytical process. However, when these assumptions deviate from reality, the consequences can be severe.
Take for example the Black-Scholes model used for pricing options. It assumes that market movements follow a geometric Brownian motion and that markets are always efficient. In practice, we know that markets can behave chaotically, influenced by human emotions, external shocks, and economic cycles. If practitioners latch onto such models without questioning the underlying assumptions, they’re setting themselves up for disappointment.
In finance, a common pitfall is assuming that past behavior can predict future outcomes. A classic example can be seen in the 2008 financial crisis, where many relied on historical data to forecast mortgage defaults. As we learned, the models didn’t adequately account for extraordinary market shifts.
To mitigate some of these limitations, practitioners can:
- Regularly update models based on new data to reflect evolving conditions.
- Use a variety of models to triangulate the most probable outcomes.
- Incorporate expert judgment alongside quantitative data to address assumptions.
In summary, challenges in understanding randomness coupled with the limitations of model assumptions can significantly impact the utility of stochastic modeling in finance. Investors must remain vigilant and adaptable in incorporating these insights to navigate the complexities of the stochastic landscape.
Future Directions in Stochastic Research
As we delve into the landscape of stochastic processes, it's essential to consider the frontiers of this field. Future directions in stochastic research not only illuminate potential advancements but also reflect the evolving needs of various industries. By exploring these new territories, we can harness emerging methodologies that may significantly enhance our understanding and application of randomness in decision-making.
Emerging Technologies and Innovations
The rapid advancement of technology is reshaping how we understand and utilize stochastic processes. Innovations have introduced tools that make complex models more accessible and applicable. For instance:
- Machine Learning Algorithms: These algorithms are adept at processing large datasets and identifying patterns. By integrating stochastic models with machine learning, we can create predictive models that adapt in real-time. This helps in more accurately forecasting financial markets, which are inherently unpredictable.
- Quantum Computing: Though still in its infancy, quantum computing holds the promise of processing information at speeds that classical computers cannot match. Stochastic algorithms designed for quantum systems could lead to breakthroughs in various areas, such as financial modeling and risk assessment. The quantum realm could redefine how randomness and probabilities are approached.
- Big Data Analytics: The coming together of big data and stochastic research opens doors for new analytical methods that capture intricate behavioral patterns across different sectors. By analyzing vast amounts of data, researchers can develop more robust models that account for various uncertainties in real-world applications.
The marriage of these technologies with stochastic processes does not come without challenges. It's vital to consider the implications of these innovations, such as:
- The need for regulatory frameworks to ensure ethical use of technology.
- The importance of transparency in algorithms used for predictions, especially in finance.
"Innovation in stochastic processes isn't simply about new models; it’s about finding ways to make these models more applicable and user-friendly in real-world situations."
Interdisciplinary Applications
Stochastic processes are not confined to finance or economics; they cross the boundary into diverse fields, creating a tapestry of applications that can enrich our understanding of complex systems. Interdisciplinary research is increasingly vital for adapting stochastic methods to various domains.
- Healthcare: In medicine, stochastic models are used to predict the spread of diseases and the impact of treatment strategies. For example, epidemiological models, like those used in studying COVID-19, rely on stochastic processes to account for random variations in disease spread.
- Environmental Science: Stochastic modeling assists in predicting weather patterns or assessing risks related to climate change. By understanding the randomness inherent in environmental systems, planners can devise more effective response strategies.
- Supply Chain Management: Businesses incorporate stochastic processes to optimize inventory levels by predicting demand fluctuations. This helps reduce costs while maintaining customer satisfaction by ensuring products are available when needed.
- Sports Analytics: The application of stochastic models in sports is another fascinating area. From player performance predictions to game outcome analyses, these models provide insights that influence coaching decisions and betting strategies.
By expanding research into these interdisciplinary territories, we not only broaden the impact of stochastic processes but also draw on collective insights that can drive significant advancements across multiple sectors. Emphasizing collaborative approaches can lead to richer understanding and innovative solutions to complex challenges.
The End
The conclusion of this article underscores the crucial role that stochastic processes play in multiple domains, especially in finance. By weaving through intricate details and practical applications, the text elucidates the inherent complexities and benefits of understanding randomness and variability. Insight into stochastic processes not only empowers investors and finance professionals but also equips them with tools to navigate uncertainty.
Recapping Key Insights
In reviewing the preceding sections, several key insights emerge:
- Foundational Principles: Stochastic processes are fundamentally rooted in probability theory, blending randomness with structured patterns. This dual nature allows analysts to develop models that can predict various outcomes despite inherent uncertainties.
- Diverse Applications: The applications of stochastic models extend beyond finance into fields such as engineering, economics, and risk management. Each application showcases the versatility of these processes in interpreting complex systems.
- Model Limitations: Understanding the limitations of stochastic models is just as vital as grasping their advantages. Model assumptions can significantly impact the reliability of predictions.
These insights form the backbone of the discussion around stochastic processes, helping clarify their importance in today’s analytical landscape.
The Importance of Stochastic Understanding
Grasping stochastic processes is no minor feat. This understanding holds significant implications for multiple stakeholders:
- Investors: For investors, a deep dive into stochastic models translates into enhanced decision-making skills. Knowing the probabilities and potential fluctuations can lead to better risk management.
- Financial Analysts: Analysts benefit from using stochastic methods to price options or assess portfolio strategies. Such models add robustness to their analyses, helping them present sound advice.
- Educators: Teaching stochastic concepts encourages critical thinking in students. It fosters the mindset of approaching problems with a balance of analytical rigour and creative consideration.
- Students: For learners, gaining insight into stochastic processes prepares them for future careers in data-heavy industries. The ability to decode randomness can open avenues in banking, research, or even tech.
"Stochastic processes are the bridge between chaos and predictability in our decision-making frameworks."
The journey through stochastic processes has profound implications for our grasp of complexity. This knowledge is critical not just for academic interest but for real-world applications, making it an invaluable asset for today’s thinkers and doers.